Imagine you were building software in the 1990s: Users receive your software as a binder of 12 DVD-ROMs. Once they install it (which takes about 3 hours), it runs locally on their machine because the internet is neither fast nor ubiquitous yet.
The only way you could find out what users like, dislike or want is by dispatch people to watch users use your product—which is expensive and, more importantly, weird and creepy. Sure, you could also ask them, but self-reported data is sketchy (yes, I always use 2-factor authentication).
Examples like the above should make us grateful for the wealth of data we have these days. Today, product usage data is easy to come by. You can almost watch users edit and navigate in real time. Aggregated, this data lets you make quick decisions about features to build, settings pages that need revamping and other useful things.
With renewed gratitude and awe of the product data we get to have these days, let's dive into how to make the most of your data!
What is product usage data? At the risk of stating the obvious, product usage data is any measurement of how your SaaS product is being used.
Product metrics are a gold mine for product insights: monthly active users, feature adoption rate, good conversion rate. Traditionally, companies used surveys and study groups to understand what active users care about, to explore user behavior, and to experiment with new product features. While still a valuable input, the traditional methods are expensive, limited in scope, and sometimes produce different user behavior than what occurs naturally. With more modern data analytics, it is now possible for a SaaS company to collect data on what users are actually doing in their products without any intervention.
The concept of "data-driven decision-making" has been very trendy for the past decade or so, and product metrics are the key to making this a reality. The right metrics inform where to invest engineering/product/design (EPD) efforts, how to resolve customer satisfaction issues, and even how to upsell more customers. Ultimately, product metrics can help any product better shape its product strategy.
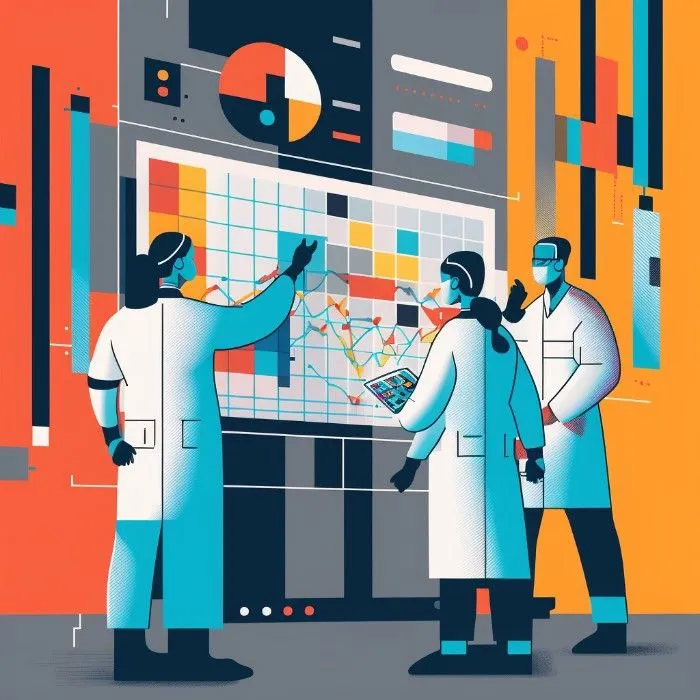
What product usage data is not
Some guides mistakenly refer to various figures as "product usage data." Some of the most common examples are product performance metrics or product adoption metrics, like net promoter score (NPS), customer satisfaction score, etc. Business metrics are another common perpetrator, like customer acquisition cost (CAC), monthly recurring revenue (MRR), and customer lifetime value (CLV). But neither tell you how your product is being used. Both customer satisfaction and financial figures are undoubtedly important and are related to product usage data but should be excluded from the definition of "product usage data."
Examples of product usage data
While unexciting, there's a reason these product usage metrics are so frequently used by product teams -- they are simple and valuable. If you have a product, you should know these numbers.
Active users
Active users are most commonly measured on the time horizon of a day and a month. Daily active users (DAUs) and monthly active users (MAUs) are the number of users that use your product during a specific day/month. Some consider DAU and MAU to be a "vanity metric" for a CEO to flex their growth on Twitter or LinkedIn, but they are essential for any product. At its core, active user count gives you a bird's-eye view of your product. When your number of active users increases, your product is doing well and can be used to identify and measure power users, for example. When your number of active users decrease, something is likely going wrong (or it's just a holiday lull). Useful for a higher-level pulse check, active user counts do not produce targeted conclusions, since they are often influenced by various other factors (e.g., customer retention, marketing spend, product/feature launches).
Churn rate / retention rate
Churn and retention are two sides of the same coin. Churn rate is the percentage of users that stop using your product during a given time period (e.g., during the user's first week/month). Retention rate is the percentage of users that continue to use your product after a given time period. High churn and low retention are signs that you need to help new users understand your product's value proposition faster and get them to an aha moment.
Feature adoption rate
The percent of customers that used the target feature during the time period. Feature adoption rate can help your team pinpoint which features need more love and which are succeeding. Sometimes in-app messaging is all it takes to increase feature usage – your users simply do not know the feature exists or how they should use it. In other cases, core functionality might be missing. User session playbacks and user event data can inform the appropriate antidote.
Usage frequency
Usage frequency can be measured on two extremes, as well as everything in between.
- Broadest -- for the entire product: How often are users using your product?
- Most specific -- for a target action (e.g., creating a document): How often are users taking the target action?
Generally speaking, you want users to be engaged in your product, so you want to see higher usage frequency. In some rare cases, making certain processes more efficient can actually decrease usage frequency while increasing customer satisfaction.
Time-to-value (TTV)
Time-to-value measures how long it takes a user to reach one of your product's "aha" moments. For Slack, this is measured as the time it takes a workspace to reach 2000 messages. Decreasing TTV is a surefire way to improve the new user experience and convert new customers. If your TTV is high, consider:
- Enhancing your product's in-app messaging strategy with onboarding checklists, contextual tooltips, and announcement modals.
- Productizing "happy paths" (e.g., getting started flows) to make it easier for new users to discover your app's killer feature(s).
Error/bug rate
Of all the metrics, error/bug rate is the most technical. It might take more effort to set up the right data capture here, but bug rates are critical. There are various ways of measuring bug rate, but the most common are:
- Number of bugs for a product/feature over a period of time
- Percent of actions in a product/feature that result in a bug
- Percent of users that hit a bug over a period of time or across a number of sessions
Understanding how often bugs occur relative to usage can be vital for improving the customer journey and increasing customer loyalty. While bugs are inevitable if your team is shipping quickly, there needs to be a balance in quality. Otherwise, you risk user churn.
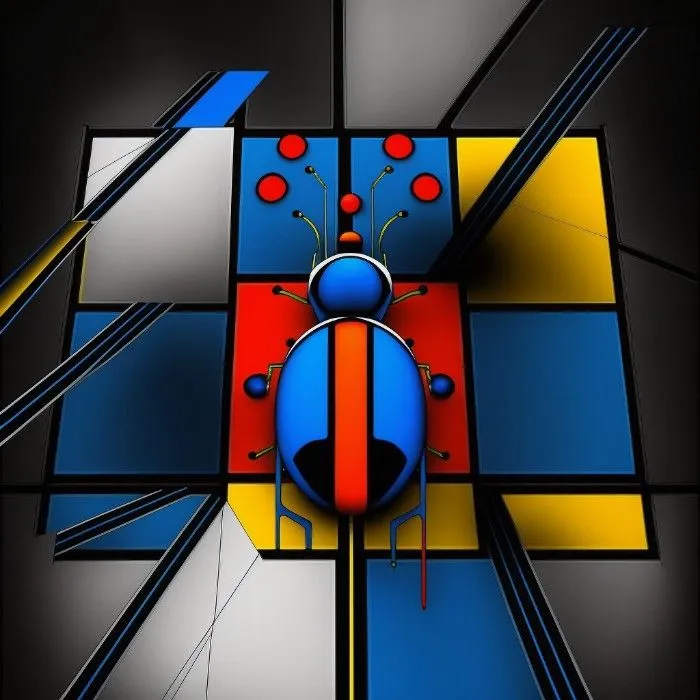
Contextualizing product metrics
Across each of the metrics in the previous section, you might have noticed each metric did not have an exact definition. It is important to choose product metrics and a product metrics framework that makes sense for your product. Here are a few examples:
- Time: The component of "time" is variable for active users, churn/retention rate, feature adoption rate, usage frequency, and error/bug rate.
- User profile: You can slice each of the product metrics above by user profile: new vs. old, region, age, primary use case, etc.
- A/B test: Compare two data sets where all but one (or a small number of) variable is the same. A/B testing is commonly used to understand how a specific feature impacts the user experience -- set A for the normal app data; set B for the app where the feature is on/off.
- Other factors: For some products (e.g., TurboTax), users are expected to only appear once a year. Their team cares more about product usage metrics like completion percentage, time to completion, and click reduction.
These examples of contextual layers underscore how important it is to make sure your product metrics fit your product. The "wrong" product usage metrics, like choosing the wrong key performance indicators (KPIs) for a team, can result in wasted effort. The "right" metrics lead to better prioritization and business success.
Why product usage data is important
Product usage data is useful for many teams, including, but certainly not limited to, the ones highlighted below. Integrating product metrics across your organization leads to better decision-making. All teams benefit from objective data, especially as they scale and the number of subjective opinions increases.
Product team
Obviously, product metrics are fundamental to product teams. Equipped with the right product metrics framework, a product manager can understand the entire customer journey – from the new user experience to power user experience.
Product engagement metrics, like feature adoption rate and feature usage rate, can help product managers more effectively prioritize EPD efforts. For example, the latest product usage analysis reveals that a feature has high adoption for new users, but low feature usage for existing users. This might indicate that users interact with and discover the feature, but do not understand its value. Product managers might then look for wins with informational tooltips, templated creation flows, or functionality improvements to better help customers meet their business goals. Or sadly, it might be a feature you need to unship.
Customer success team
A Customer Success (CS) team cares about:
- Engagement metrics -- like feature usage
- Average customer metrics -- like average customer lifespan
Understanding customer behavior allows a CS team to tailor more useful content to clients. For example, say a customer is heavily using feature A but not using feature B at all. In future interactions with that customer, it makes sense to highlight enhancements to feature A and how feature B could help them get more out of the product. Data-driven decisions like this can propel a company to reduce churn rate and improve customer loyalty. Moreover, using a strong Airtable alternative can enhance data management and provide deeper insights into customer behavior.
Sales team
Like the Customer Success example, engagement metrics can be critical for an Account Executive. Knowing which members of a prospect organization are highly engaged in your product can make it easier to find a product champion. Similarly, real-time insight into features usage empowers your Sales organization to drive more useful and relevant conversations with prospects. In the long run, deploying product metrics in Sales and CS raises your average customer lifetime value.
Growth team
Growth teams can also use product metrics to make better decisions. There are a number of primary use cases on the Growth side:
- Marketing campaigns: Knowing that your users respond best to a certain in-app call to action (CTA) can influence the CTA for a new product/feature launch.
- User targeting: Identify trends in product usage by user type to pinpoint which users should be targeted for paid ad spend. Target user information can also be used to decide on ideal marketing channels.
- SEO: Prioritize search terms to target based on the features that new users are adopting and engaging with the most. It's probable that prospective users would find value in the same use cases.
Embedding product metrics in your team's growth strategy produces more efficient growth efforts: increasing new customer growth rate, decreasing customer acquisition costs, and improving market adoption.
How to use product usage data
When product metrics are collected accurately and made easily accessible, it is just a matter of time before they work their way into everything your company does. The previous section briefly mentioned a few examples of how you can use usage data to inform decisions. Below you'll find three in-depth examples of ways that your team can turn product metrics into product actions.
Feature launch
Scenario
To better illustrate this use case, let's say your team has decided to launch a new feature that allows users to use GPT-4 inside your product.
Product metrics usage
How does the team arrive at this decision? Customer feedback data includes requests for this feature. However, the team is well-equipped with their product's usage data and already has a clear sense for what users want.
- Search data: Combing through users' search results within your app's command palette, your team finds many hits for "ChatGPT" and "AI". This raw search intent data is a massive signal for user intent.
- User onboarding: Your user onboarding flow has a question "What are the primary reasons you are trying our product?" You are seeing an uptick in the number of users that are selecting the AI-related option.
Now that the feature has been launched, your product managers are wondering how they can improve the feature. A few key metrics help the team hone in on a few targeted improvement areas.
- Feature discovery rate: Only 10% of users reach the URL path for the new feature. It's clear that more needs to be done with in-app messaging to nudge users to check out the feature.
- Feature engagement duration: Of the users that check out the new feature, only 25% actually use it. Considering the current UI/UX, the team decides to add templated "Getting started" questions/prompts and some light animation to make the feature friendlier and easier for users to engage with and reduce user friction.
Value add
Using product metrics in this scenario has allowed the team to make significant decisions with high confidence:
- Launch a new feature that users care about
- Improve the feature in targeted ways to (a) help users discover the feature and (b) get value from the new feature
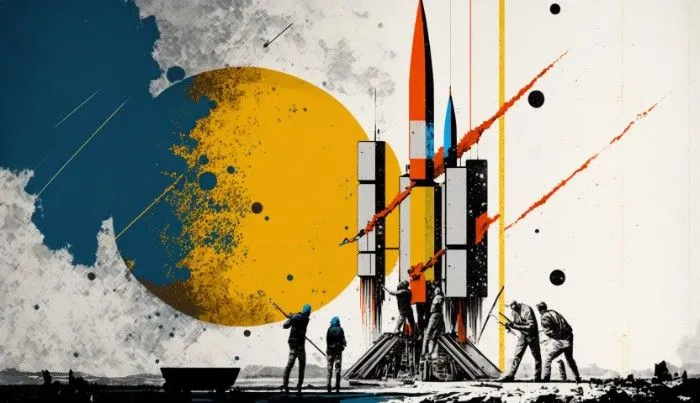
Pricing tier refinement
Scenario
The Sales team for a B2B SaaS product -- let's say a website-builder tool, like Webflow -- has been considering adjusting their pricing structure.
Product metrics usage
From customer and prospect calls, the Sales team suspects that the free tier is too generous. Looking at their overall usage data, they can confirm this.
- Upgrade rate: Less than 5% of free tier customers ever upgrade to a paid subscription.
The question then moves to how the pricing tiers should be adjusted.
- Paid tier feature usage: Many of the features gated to paid plans are not being used by paid accounts.
- Free tier feature usage by customer size: Overlaying feature usage data with the size of the customer's company reveals that within the top 30% of free tier customers (by size), most customers use the CMS and custom styling features. Smaller free-tier customers are using these features at a much lower rate.
From the findings above, the Sales team proposes two major changes for new customers:
- Make CMS and custom styling paid features.
- Migrate the unused paid features onto the free tier.
Value add
After implementing the pricing changes outlined above, more new customers start using your product on a paid plan, and more free-tier customers are upgrading to a paid plan. In turn, your average revenue per customer is on the rise.
User onboarding
Scenario
A few months ago, the team added user onboarding flows to help users find your product's two main features.
Product metrics usage
The team isn't sure whether these flows are improving the product experience. Your product managers dive into usage data on an A-B test format (before/after the user onboarding was introduced) and uncover some key takeaways.
- Churn rate: The onboarding flow has had little to no impact on churn.
- Upgrade rate: The onboarding flow has had little to no impact on churn.
- Feature usage rate, in an A/B test format: Here's where things get interesting. Looking at the feature usage rate for the two features targeted in the user onboarding flows, it is clear that many more new users are discovering and trying the two features. That is awesome! Unfortunately, only 30% of users use these features after first trying them.
The top-level metrics, like churn and upgrade rate, have not changed. However, the onboarding flows have been effective at driving attention to the important features. The product challenge is making the features stickier.
Value add
Instead of wasting time on solving a problem that doesn't exist ("fixing" the user onboarding flows), the team knows where to prioritize their efforts. EPD changes are now more likely to change important metrics, like reducing churn.