Ever since I was young, I've always enjoyed filling out surveys and giving feedback to customer support agents over the phone. There's nothing like being asked to complete a four question survey after waiting for an hour and a half on hold to get one question answered!
I joke, but it is true that as I've worked more in software and product, I've become more inclined to respond to quick and well-structured requests for feedback.
That’s because I know that collecting high-quality feedback and turning it into better product decisions is essential to building a great company. You need your customers' input to drive long-term PMF. I like to support companies in that effort!
However, it can be hard to collect great feedback — getting folks to respond to surveys and offer their opinion is hard.
What can be even harder is turning all that feedback, as well as all of your other user data, into actionable insights.
You face two primary challenges:
- Collecting accurate and comprehensive feedback data
- Turning all of that data into actionable insights through discussion and execution.
A lot gets written about #2, but this assumes that you have the best quality data. Few companies are generating the best type of user intent data they could be, because they aren't giving users an incentive to provide it.
But we've discovered a new way to improve your feedback collection process, one that creates a more holistic feedback management flow.
A reliable user assistant, like our Copilot, is the best way to capture raw intent data. This is the best basis from which to improve your product, marketing, and positioning.
We've found ways to helps our customers incentivize their user base to provide feedback, and gather intent data automatically, through Copilot’s role as an AI user assistant. It solves problems, collects tickets, and provides better, highly accurate, in-the-moment user intent data. Let's dig in!
What exactly is feedback management?
Feedback management is designed to help you create a better product and a better business. It gives insight into your users' wants, needs, and desires and allows you to improve your product marketing and competitive position.
Feedback management has several core goals: to create a better product, better usability, and better marketing that aligns with the messaging customers send about their wants and needs. The other is to develop a better overall business stance that allows you to drive more revenue and out-compete your peers.
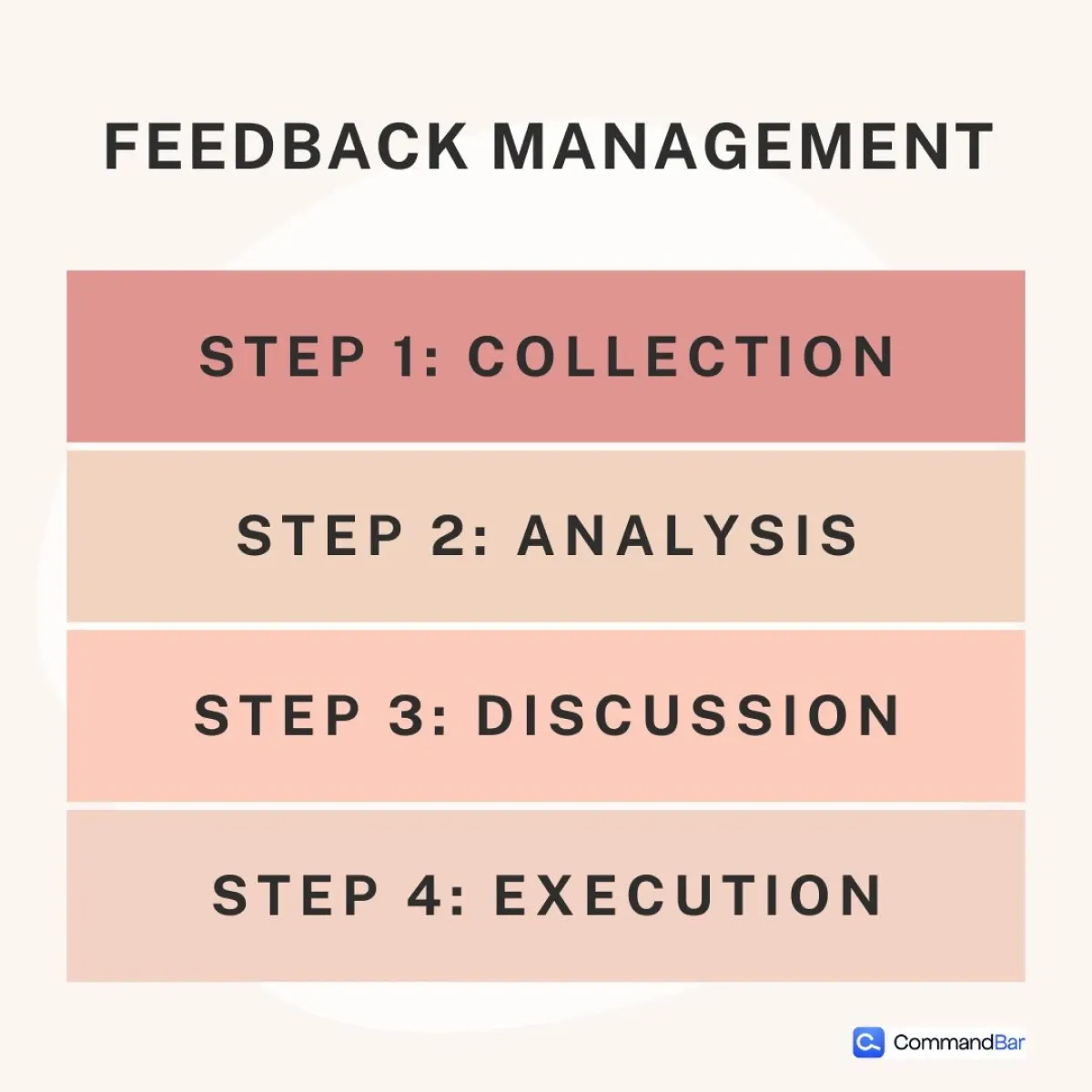
More directly: the process through which you turn all kinds of user feedback and intent data into actionable insight, and finally into better product.
This involves four steps:
- Collection
- Analysis
- Discussion
- Execution
Why does having an effective feedback management system matter so much? Without it, you risk not collecting feedback well, and even if you do, you may not turn it into actual insights and product improvements. To ensure that you continue to meet the Market's expectations and satisfy your users, you need to have this system in place. It will allow you to better retain your users and reduce churn, and also identify opportunities for upselling. It can also give you a better sense of where your marketing messaging and positioning is off, or points of strength to emphasize
I don't think any of this is revolutionary for you to read, but it can still be hard to wrap your head around how to create a simple but effective feedback management system. That's because there are some distinct challenges that you may face.
Challenges in collecting feedback
However, there can be challenges in collecting great feedback and creating a smooth feedback management system.
First, we've got challenges with data collection. Not only is it hard to get users to engage with your surveys and provide feedback, but even when they do, you face other biases like non-response bias or the power user bias.
While these are not insurmountable obstacles, if unaddressed, they can lead you to very biased and unhelpful feedback and data.
Even the best feedback management system will not allow you to drive accurate product insights if your foundational data is poor. So, finding ways to incentivize your users to give you feedback and engage with your surveys is critical. You need to meet folks where they are and find a feedback collection flow that works for everybody. How do you do that?
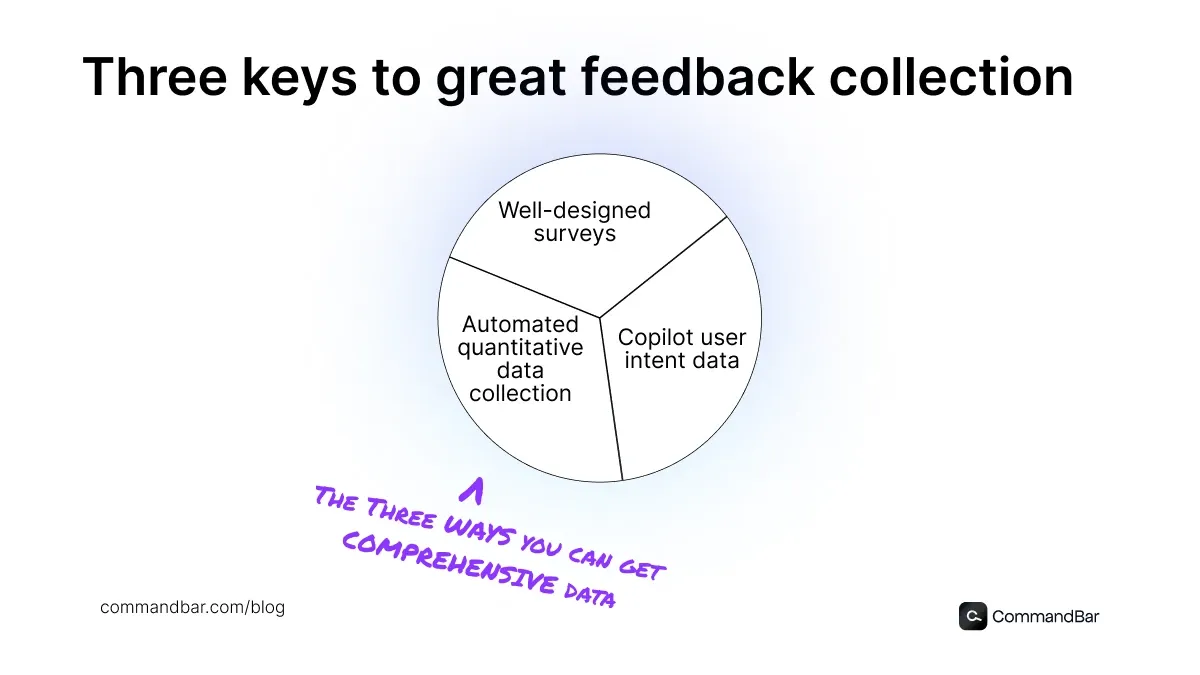
Improving feedback collection
It's important to mention that standard feedback collection can be improved through better incentivization and survey structure. I just wrote much about this in our non-response bias article, but let me briefly review some key strategies you can use to improve your feedback collection and, thus, your feedback management protocol.
The first is to have clear, concise, and targeted surveys. You want your surveys to be as short as possible, with clear context and questions, and to only ask them of the right people.
Second, you want to have clear incentives for your users. Ask yourself why users should take time out of their day to answer their questions. That's why some folks offer a specific monetary reward, while others don't go that far. Instead, they offer a prompt or a priming for why folks should get feedback.
They say: “we want to improve your experience. We want to make things easier for you. We want to get better to better serve you. And that's why you should answer X, Y, and Z questions.”
That allows you to create a user who is a bit more motivated to answer questions and generally primed to contribute because you have appealed to their self-interest, which, like it or not, is the core mechanism through which folks get interested.
A unique way to optimize your feedback management system
We found another interesting way to enhance the feedback management system. This involves getting raw user intent data, which you then turn into actionable insight. Let's discuss this in depth.
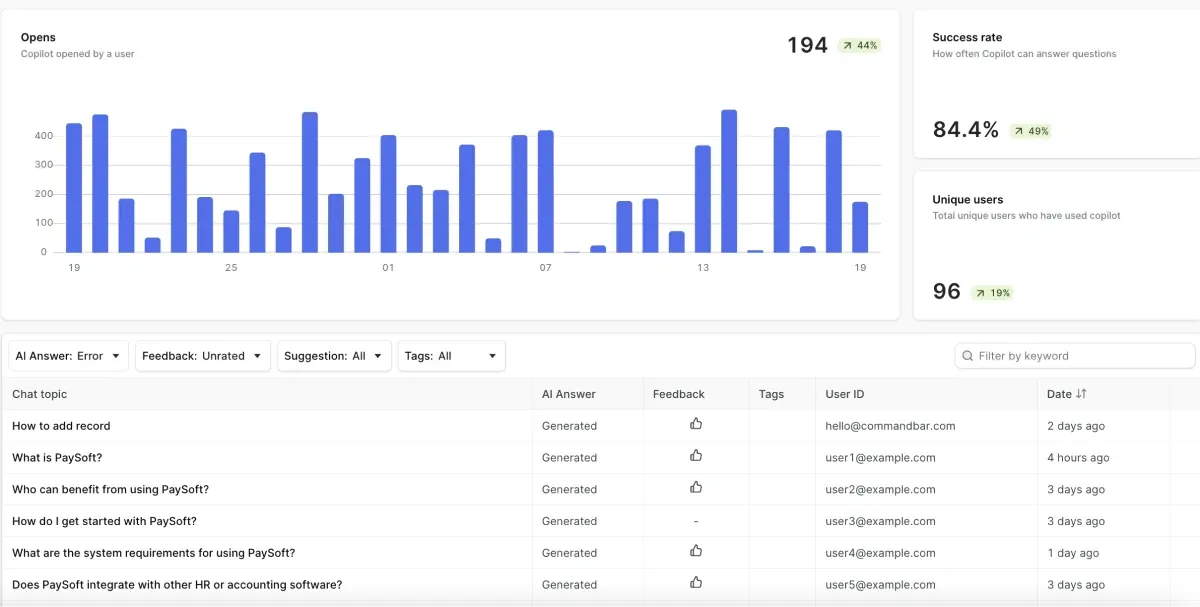
Using an AI agent user assistant
This can be accomplished through a robust AI agent user assistant. It not only guides your users through the product, deflects support tickets, and can launch in-product experiences but also offers you a huge amount of raw user intent data.
You might be asking how this differs from the feedback you normally collect from chat transcriptions or other chatbots.
The feedback you get in Copilot can not only help improve the model but can also demonstrate specifically why folks are getting frustrated, hitting dead ends, or having other issues. It ultimately guides you towards better help docs, support flows, and products overall. That's powerful!
Benefits of Copilot
When we built our Copilot, we thought of it primarily as a user support agent. It allows folks to get quick answers to questions, give in-context and timely responses, and pull from your help documentation. We quickly saw our customers deploy this as a ticket deflection tool, which has served these dual purposes well.
But what has quickly come to bear is a reality in which all of this data also serves as a huge collection of user intent information. It allows you to get a highly clear and contextual picture of what your users are trying to accomplish, how both responses or solutions work (or don’t.) Generally, it helps you better understand your user intents and how your responses manifest into emotion and action.
The chatbots of old could certainly attempt to answer some questions and potentially deflect tickets. But Copilot allows you to dynamically pull from help documentation, launch in-product experiences like product tours and nudges, and gives you a dashboard to identify dead ends and fallbacks and analyze your shortcomings.
A note: it's important to take all of Copilot's user intent data and compare it and contrast it with the direct feedback you've received through surveys and micro-interactions, as well as your quantitative metrics.
Step 2 — Analyzing feedback
The next challenge is how to coordinate your team and organize a mentality of collaboration, discussion, and decision-making based on all this data. Even with all of this user intent data, quantitative data, and feedback from surveys, the picture is not always clear. You still have to make analysis and critical decisions.
So it's important that you first align with your team on what matters, what metrics, what points of feedback, and what areas of feedback are important to your business and will cause you to make changes. How do you measure success or failure? What is a good score for each specific survey?
All of these things need to be thought about ahead of time. You can't just measure, measure, measure without a set defined system for how you intake and evaluate feedback. If you don't do this, what happens is that you have more data than you can handle and no clear criteria to assess how you're doing. If things aren’t obvious, you risk getting lost in discussion, debate, and frustration between your team and the proper course forward.
Processing feedback data
Once you've collected all this data, you still face a challenge in fully processing it and turning it into actionable insight. Let's discuss some best practices to minimize friction and maximize the helpful data you get from these different surveys, data points, and more.
The first is to distinguish between qualitative and quantitative data. I've written about this in the past: you need different tools and mindsets when you compare both kinds of data.
For your quantitative data, you want to set actionable and specific KPIs you seek to hit and then work towards maximizing and optimizing those. If you don't set benchmarks and goals in the first place, it's hard to assess how you're doing. You want to ensure you have a robust data analytics tool to intake, store, and help you analyze and visualize all that data. You can use specialized data collection tools or coding solutions, including Python to collect feedback through web scraping. In the latter case, you can even take a Python Screenshot to document and review your data collection process.
On the qualitative side, it's also important to set clear goals and benchmarks, though because you're dealing with written feedback, it can be a bit harder to set universal goalposts.
Across both kinds of data, what you're looking for are clear patterns.
Are there areas where you consistently see weak scores or negative feedback qualitatively and other patterns and trends? This helps you prioritize different sections of your feedback and digest them for your team.
Tools for collecting feedback
Let's talk about what kind of tools you can use to effectively collect feedback.
We've already discussed how Command AI's Copilot can help you improve your collection process and unlock a new level of data. However, you still need a baseline tool that can manage all of your analytics, like Amplitude or Heap, as well as a background data collection tool like Hotjar, which can record session recordings and collect other heat-mapped data.
Then you also need tools to effectively intake and store all of your feedback, and this can range from something simple like a Kanban board to something very complex like a very well-built-out Notion database. Whatever it is, you want to arm yourselves with the appropriate tools for your company's size and needs.
The needs of a small startup will be very different than that of a large enterprise company because of both the size of the data but also the complexity that might be in the feedback.
You also want to remember that as your business grows and your feedback grows, you want tools that can scale with you so make sure that however you're collecting data through surveys, quantitative data management tools, and other analytics tools, they're able to be fairly dynamic and scale with you as you grow.
Step 3 - Delegate and discuss
Another important aspect here is understanding who within your team is responsible for collating all of this data and running analyses. This may have to be a founder if you're a small team. But suppose you're a larger startup or enterprise-level company. In that case, you may have dedicated folks in your product team who you can assign as your feedback management lead.
This means that, as part of their role, they need to understand all of the different channels through which you are collecting feedback. They should put together clear summaries and analyses of different channels of feedback and then lead and facilitate discussion between stakeholders on this feedback. They help create the proper feedback management system that you need for successful product changes and decision-making.
And that's hard to do. So it's important to hire not only someone with the product and technical chops to understand and break up the data but also someone who can manage the conversations, immersion, and priorities of different stakeholders.
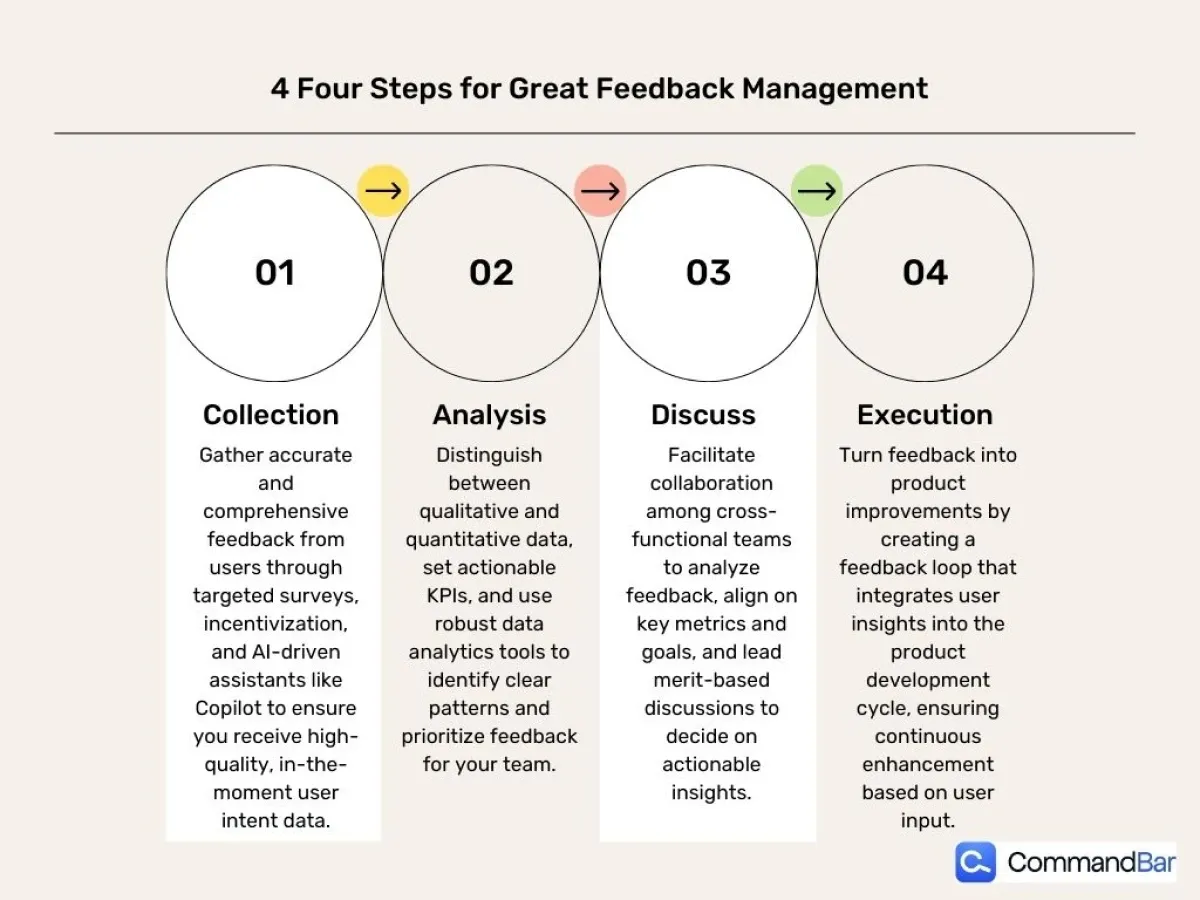
Step 4 - Prioritizing feedback and executing a plan
It's important to understand how your team will prioritize different levels of feedback. I like to be simple and use three distinct levels: high, medium, and low. Additionally, you might have it on a backburner or a rainy day list.
While these are generalizations, each company is going to have a unique user base and product that will move certain issues up or down this priority list.
Let's walk through these:
High priority
High-priority issues are mission critical, affect many of your users, or are causing acute and immediate problems. This might be a bug, a broken feature, or something to do with your system administration. Whatever it is, this is a universally accepted high-priority item that needs to be dealt with immediately. These are the easiest to identify, though they may be the hardest to solve.
Medium priority
A medium-priority item might affect fewer users, but it is still important. While the product is still working, specific features or parts of it might be broken and need to be fixed. This might not be something that you need to immediately fix, but it should be added as a priority item to the roadmap and dealt with fairly soon. This could be something to do with small bugs or general usability improvements.
Low priority
Low-priority issues might be limited in scope, size, or importance or only affect a smaller number of users. They can be added to your roadmap but as non-priority items. Finally, you might have some feature requests or other UX requests that are not priority items but that you consider as you begin to develop a longer-term roadmap.
In addition to prioritization, you must ask:
Who owns this feedback management process, what channels do you look at, how do you do a meta-analysis, how do you communicate with your product and engineering teams, and what is the actual feedback evaluation process?
Once you've taken in all of this data and applied this clear analysis to it, you still face another challenge: creating a feedback loop through which this data turns into insight, which turns into discussion, which turns into better product and better improvement. This is that feedback management loop. How do you optimize this?
Well, there are a couple of best practices.
First, it's important to clearly communicate the insights to your team. Digestible, concise, concise presentations filled with data and understandable are key.
You want to ensure that all of your stakeholders are driven toward a merit-based, good-faith discussion that does not depend upon their biases or specific product areas.
Once you have this brutal discussion and get input from different stakeholders, you want to set clear expectations for decision-making.
How will we decide whether to incorporate the feedback into the product roadmap and turn it into actual product changes?
You need continuous feedback and want a fully integrated loop into your product development cycle.
Hopefully, you're looking at this feedback and discussing it regularly. That's what we do here!
Best practices for team and stakeholder management
First, ensure a clear and consistent calendar for collecting and analyzing feedback and having discussions between your teams. Find times when your product, marketing, growth, customer support, and engineering teams can all gather. This cross-functional approach can provide more valuable and diverse insight.
Having a mindset of collaboration and connection with your users is also important. It's easy, when you're looking at aggregated data, in particular, to forget that actual users on the other end are making these suggestions and giving this feedback. While your resources may not be too vast, it can still be worthwhile to get in touch one-on-one with users and have direct communication, particularly when the feedback is highly negative or positive. This can give you a clearer picture of the context and color behind some of these feedback points.
Common pitfalls and mistakes
One of the most common pitfalls and mistakes folks make when trying to create a good feedback management system is to actually make these three mistakes.
The first is ignoring negative feedback. It's easy to discount some of your negative or lower score feedback, particularly when you have a lot of highly positive feedback, but that's not healthy, right?
Even if your product has a really solid net score, there are always areas for improvement. Ignoring negative feedback, even if it's an outlier, can lead you to forget about and ignore some critical areas of your product that might later become big issues.
Another is making the feedback process overly complex.
We talked about how it's really important to gather different types of data and to have different tools to analyze it, but I don't want you to over-engineer this process. When you do that, you make it hard for users to get feedback, you make it hard for your teams to understand that feedback, and you make it hard to make better products out of that feedback.
So, the simpler this process, the better. While the first two are bad and occasionally happen, they're pretty easy to execute.
By far, the biggest mistake people make is failing to actually act on all of this feedback.
People create surveys, they collect data, and it just sits there. Or maybe they even aggregate it and share it with the team. But there might be a bit of an aversion to incorporating that feedback directly into the product roadmap.
Your team has its priorities, certain things it thinks users want, and it can be very easy to lead with a product-first mentality. However, as Steve Jobs talked about and as some of the best SaaS leaders know, it's really important to make your product decisions based on the user's feedback. Ensuring that your feedback management system turns into concrete change requires you to execute all of these.
Conclusion
You need to collect high-quality quantitative and qualitative data across the board.
You need an effective way to gather that data, analyze it, visualize it, discuss it, and then turn it into actionable insights that you can use to fuel better product decision-making.
That's not easy, but I'm optimistic you can execute it. As we gain more generative AI capabilities, particularly within a user assistant like Copilot, you'll be able to do more and more without spending hours and hours diving into user chats yourself.
They'll be able to extract true user insight through dead ends, fallbacks, roadblocks, and more and produce a better feedback management system that ultimately leads to stronger product development.
Let me leave you with an interesting final wrinkle.
There's a hidden fifth loop here, which is gauging how the changes you make based on user feedback factor into the user's experience, and future feedback.
It's kind of meta! Here, you're asking how changes based on feedback impact your following feedback. Ideally, you do want to have that final closure of the loop, which is to understand how effectively your team's ability to analyze, discuss, and execute product improvements based on feedback is working.
To monitor these, reach out to cohorts of users who requested the changes and ask them if you've fulfilled their needs. More passively, you can look at changes in CSAT scores and NPS scores over time.
Gathering feedback is a continuous process, not something you do once a quarter or once a year. With the right feedback management system in place, you can feel confident in your team's ability to continue to build features and products that people want and enjoy using.